DataFactZ’s AI Agentic Architecture empowers enterprises with adaptive, reusable agents that continuously learn and scale, driving smarter decisions and operational efficiency.
Achieve True Enterprise AI with Adaptive, Scalable Agents
Focused on enterprise-grade solutions, the AI Agentic Architecture integrates essential infrastructure, security, and compliance to support AI agent deployment. It offers enhanced access controls, real-time observability, and alignment with regulatory policies, ensuring a secure and reliable foundation for AI initiatives.
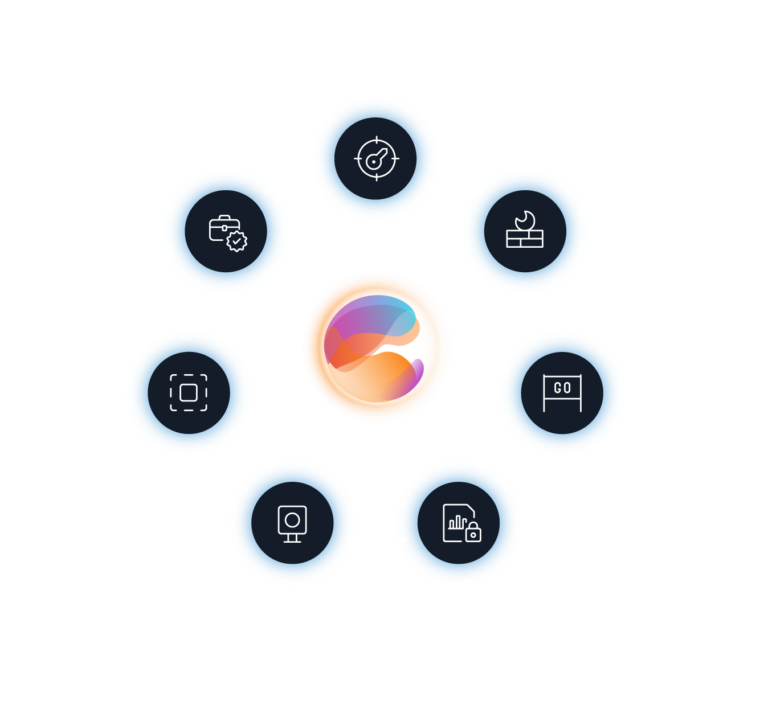
Agentic Architecture leverages different types of AI agents, each designed to excel in specific areas, from real-time responsiveness to achieving strategic goals. These agents work in concert to provide a dynamic, integrated solution that enhances your enterprise’s ability to adapt, optimize processes, and achieve both immediate and long-term objectives.
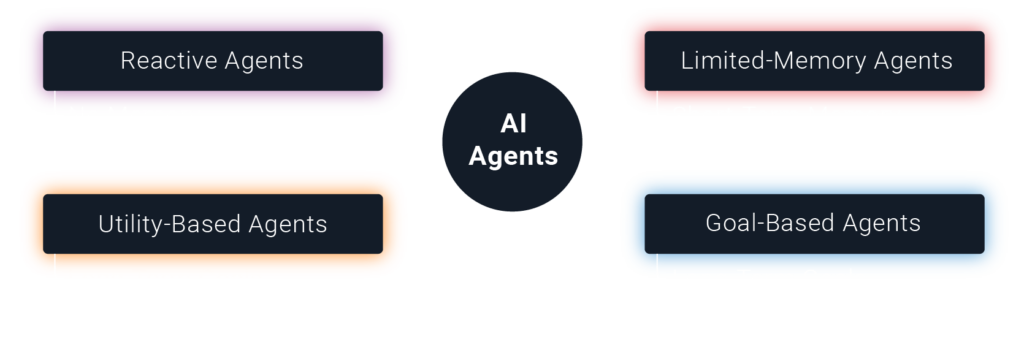
Reactive Agents respond to specific inputs or changes in their environment without storing past experiences. These agents operate on a condition-action basis.
- DB Agent: Reacts to requests for data access and integration across multiple sources without retaining any history of past connections.
- Data Quality Standardization Agent: Reacts to real-time data quality issues, detecting and correcting inconsistencies as they occur, without storing past anomalies.
Utility-based Agents make decisions based on the utility or value of possible outcomes. They assess various actions to choose the one that maximizes the desired outcome.
- ETL Code Generator: Generates SQL code based on the best way to move data between sources, evaluating the utility of different code approaches to optimize data transfer.
- Synthetic Data Agent: Generates synthetic data based on the utility of the generated data in specific scenarios, balancing factors like privacy, realism, and variability.
Limited Memory Agents can store and use past experiences to make decisions or improve their actions over time. These agents have some memory of historical data but are not fully autonomous.
- Content Generator Agent: Learns from past content generation tasks to improve future outputs, adapting style and tone based on prior interactions.
- Insights Agent: Utilizes historical data and insights to refine its ability to generate more accurate and contextually relevant insights over time.
Goal-based Agents are designed to achieve specific objectives, often planning their actions and considering the consequences of their actions to meet these goals.
- Document Knowledge Agent: Works towards the goal of extracting and comparing critical information from documents, identifying discrepancies with a long-term objective of improving document consistency and accuracy.
- Insights Agent: This agent could also be considered goal-based, as its primary objective is to generate actionable insights to aid decision-making, adjusting its approach based on predefined goals.
Enterprise AI Agents work together to create seamless workflows across business functions. By integrating these specialized agents, businesses can automate complex processes, from data quality management to content generation, ensuring that each function benefits from the strengths of the others. This collaboration drives efficiency, enhances data-driven decision-making, and ensures consistency across all operations to improve productivity across business functions.
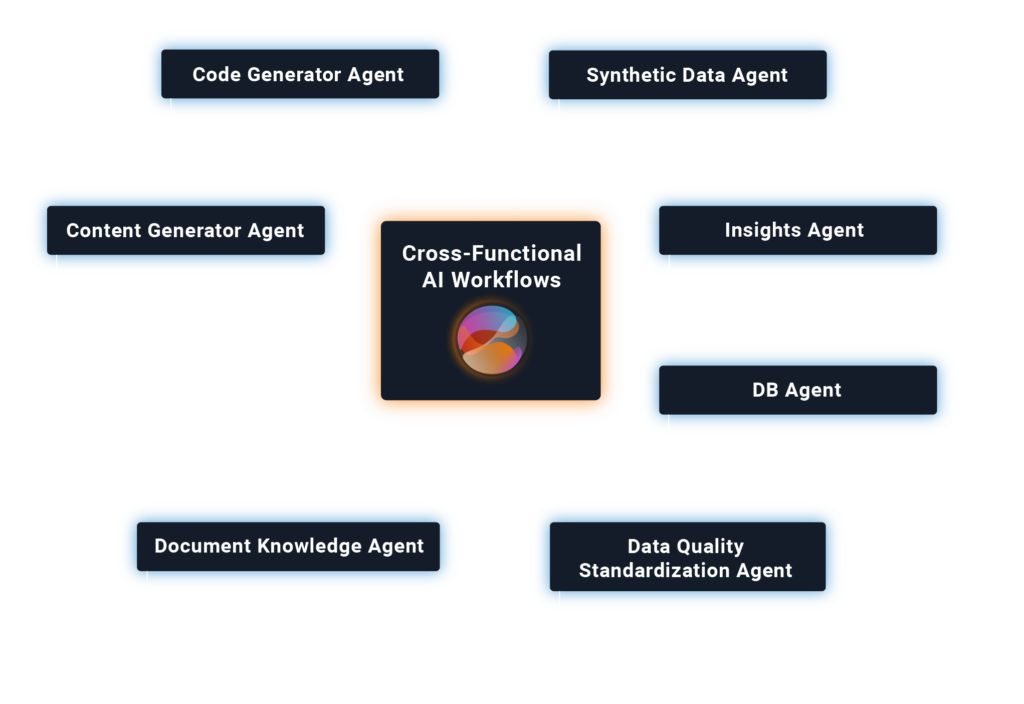