There is no AI without the right data. Less than half of AI projects make it past pilot. That’s where we come in.
The most exciting stuff in Generative AI happens when the boring stuff is optimized.
We’re your secret weapon for the boring stuff so you can do the
.
Thousands of deployments means we’ve developed proprietary templates and processes to accelerate and automate data engineering, cloud migration, devOps to BI dashboards.
How do I compete in an AI world?
Modeling techniques are being commoditized, new tools and capabilities are appearing faster than can be tracked, compute and model costs are decreasing exponentially…
Your data is your competitive advantage- genAI unlocks the power of your data, and you should build the AI apps only you can build.
The most important factors in any genAI for Enterprise or Analytics success are all determined by your data foundation.
Data Quality: Quality, well-structured data is essential for accurate generative AI results.
Semantic Understanding: Understanding the context and meaning of the data is vital. A business semantic layer establishes the meaning and relationships within the data.
Lineage and Traceability: Knowing the source and history of the data is crucial for tracking changes, identifying errors, and ensuring the accuracy of generative AI output.
Governance and Monitoring: Governance practices will affect models and output at all levels, and effective monitoring needs to to identify and rectify issues as well as enable the feedback loop.
The proper DW/ Lakehouse means you can:
- Leverage common tooling across the org by reducing the tech stack
- Enable end-to-end data governance, lineage and version control, models inherit the governance of the data
- Enable more effective monitoring
- Leverage semantic understanding and lineage for genAI output accuracy and business value
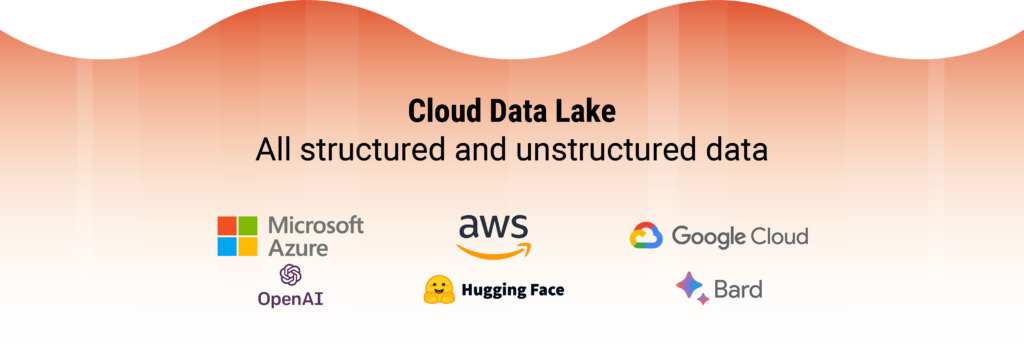
As a tech agnostic data solutions partner, we scale your capabilities, not your tech stack and costs. See how your teams can implement genAI with their current platforms and skillset>>

How will generative AI contribute business value?
Gartner identifies three categories of opportunities for generative AI:
Revenue Opportunities:
- Product Development: Generative AI accelerates the creation of new products, including drugs, cleaners, flavors, alloys, and diagnostics.
- New Revenue Channels: AI-mature enterprises see increased revenue benefits.
Cost and Productivity Opportunities:
- Worker Augmentation: Generative AI enhances content creation, summarization, code generation, and chatbot performance at scale.
- Long-term Talent Optimization: AI collaboration extends employees’ capabilities, accelerating proficiency.
- Process Improvement: Generative AI unlocks value from untapped content stores, transforming workflows.
Risk Opportunities:
- Risk Mitigation: Generative AI improves risk identification through deeper data analysis, enhancing pattern recognition.
- Sustainability: Generative AI aids in sustainability compliance, risk reduction, and embedding sustainability in decision making and product design.
What are some practical uses of generative AI?
Generative AI has numerous practical applications across various industries. Some notable uses include:
- Content Generation: Generative AI can create content for marketing, advertising, and entertainment. It can produce text, images, videos, and music, reducing the need for human content creation.
- Image and Video Editing: Tools like deepfake generators and image enhancers use generative AI to manipulate and enhance images and videos for creative and professional purposes.
- Text Summarization: It can automatically summarize lengthy documents, making it useful for information retrieval and content curation.
- Chatbots and Virtual Assistants: Generative AI powers chatbots and virtual assistants, enabling natural language interactions for customer support and information retrieval.
- Drug Discovery: In the pharmaceutical industry, generative AI is employed to design and discover new drug compounds, potentially accelerating drug development.
- Code Generation: It can auto-generate code for specific tasks, speeding up software development and reducing human coding errors.
- Anomaly Detection: Generative AI can detect anomalies in data, making it useful for fraud detection, quality control, and cybersecurity.
- Generative Adversarial Networks (GANs): GANs are a subset of generative AI used for creating realistic fake images or videos for special effects, design, and research.
- Recommendation Systems: Generative AI can improve recommendation engines by creating personalized content suggestions for users based on their preferences and behaviors.
- Sentiment Analysis: Generative AI can analyze social media and customer reviews to gauge sentiment, helping businesses understand public opinion.
- Data Augmentation: It’s used to generate additional training data for machine learning models, improving their performance.
- Medical Image Analysis: It can help with the interpretation and analysis of medical images, aiding in diagnosis and treatment planning.
These are just a few examples of the practical uses of generative AI, and the technology continues to evolve, expanding its applications across various domains.
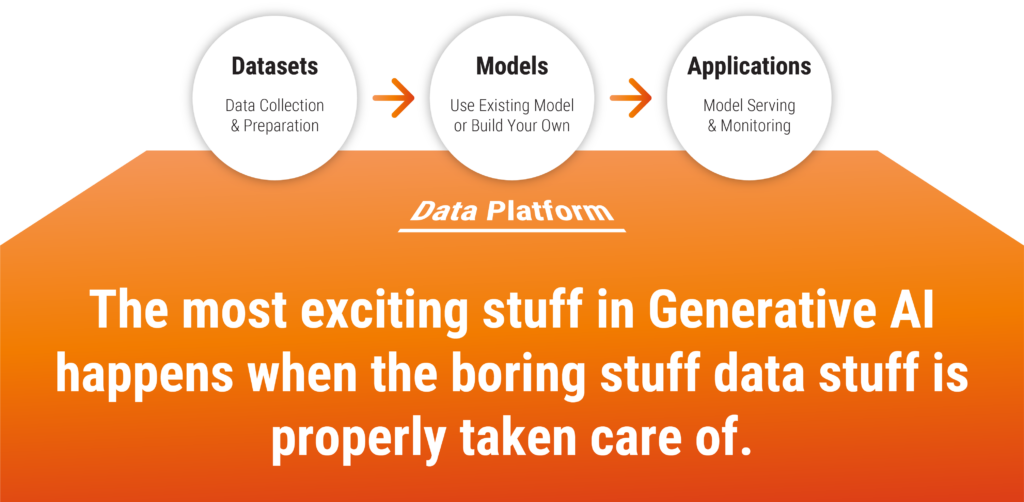
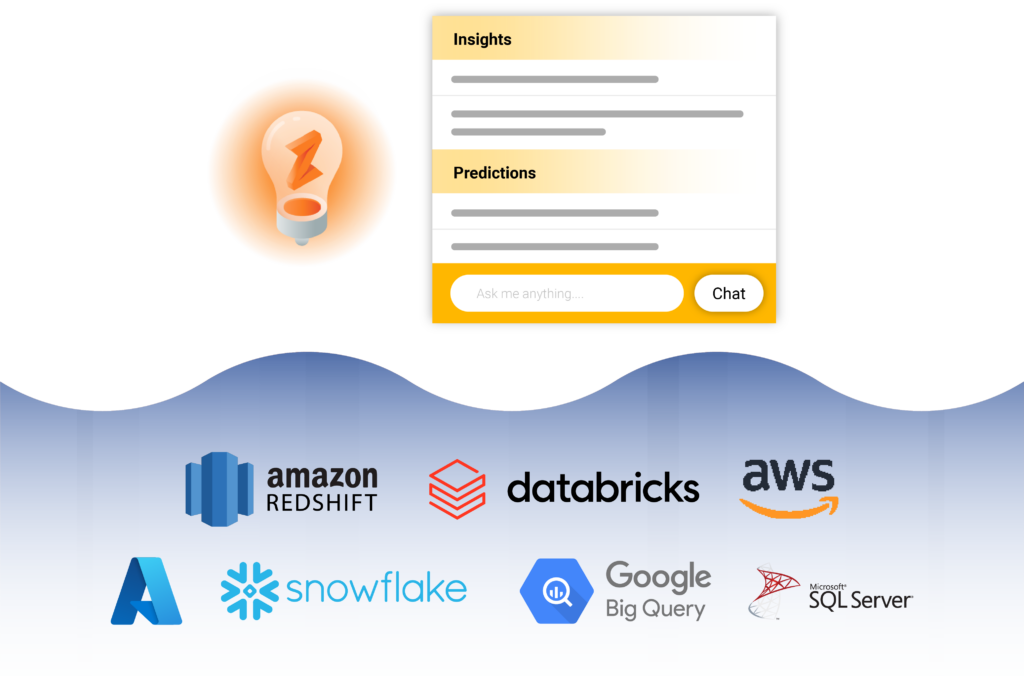