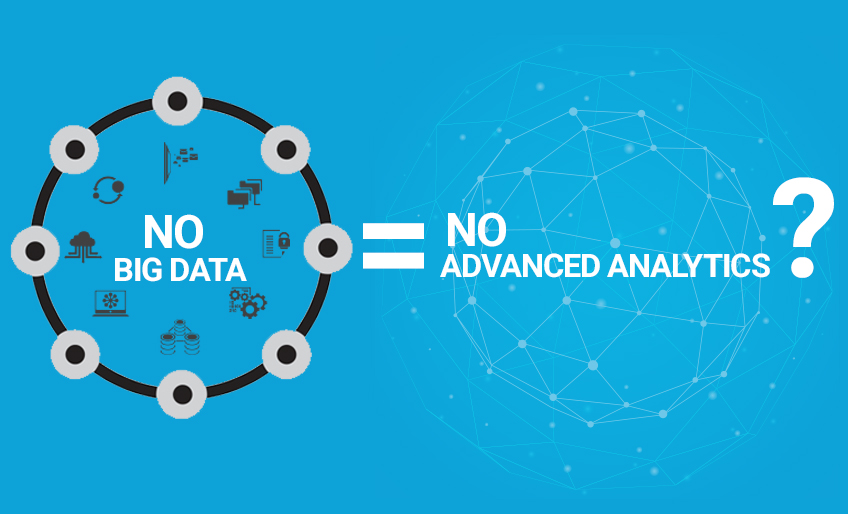
Unfortunately, we hear the above statement too often. The hype around Big Data has created a misconception that advanced analytics is not possible with Small Data, which everyone has been collecting for years.
Big is a relative term. With more and more focus on the size of data, many people are headed in the wrong direction without paying much attention to the true purpose – solving key business problems or challenges. Is this a right strategy for your business? Let’s instead focus on solving business problems first, then determine what and how much data can help us in the next step, whether it be Big Data, Small Data or Tiny Data.
In reality Big Data is nothing new, it is simply a linear extension of the historical trend of data storage getting cheaper and cheaper. Big Data has always existed – it’s just getting bigger due to the digital world and the vast amounts of data generated by machines without human intervention. What really has changed is the extent to which companies and organizations have the ability to leverage this information in order to be more competitive and successful.
Here are a couple of poor assumptions that we see in practice regularly when adopting advanced analytics.
Assumption # 1: All Data = Big Data AND Every Problem = Big Data Problem.
Wrong. Many organizations believe Big Data is becoming invincible, and seem to think it can solve every problem in every situation. We often hear “unstructured, volume, variety, zettabytes, etc.” but many times it’s not required.
In our experience, the most successful ways to leverage Big Data and show its value is no different than typical relational datasets. The primary focus should always be on understanding the business objectives, then providing an analytical solution. The data is already complex enough, there’s no reason to over complicate in the beginning with models and algorithms the senior leadership team likely won’t understand or digest. Start simple and build credibility for your advanced analytics practice.
Fortunately, Small Data – the transactional and customer data we have been collecting for years – is structured and thus more easily transformed into insights and actions.
The bottom-line is Big Data is not a panacea for all.
Assumption # 2: Small Data = Small Insights.
Wrong. In our experience, often times you will find valuable insights in Small Data.
For example, a fundamental goal of a business marketing function is to maximize the lifetime value of the company’s customers. Life time value (LTV) is usually defined as the expected present value of the net cash flows from the firm’s relationship with customers over the lifetime of the relationship. LTV is a key metric used by companies not only to measure customer equity (the sum of the lifetime values of all the company’s customers) but to also set an upper limit on spending to acquire customers. Calculating Customer LTV using Recency, Frequency and Monetary Value with a Markov Chain Model will identify the core transactional characteristics of your best customers. All of this can be simply achieved with so called Small Data.
Conclusion:
The business problem or opportunity must drive the solution. In many cases, that will involve Big Data, and in other cases in won’t. We are not against Big Data, and in fact one of the early adopters for this technology, and deeply married to every possible Big Data term that you can possibly think of (Apache Hadoop, Apache Cassandra, Apache Spark, Mongo DB, Scala, MapReduce, Kafka, etc.).
Poor assumptions are keeping organizations away from realizing the power of Small Data, and most importantly the benefits that come from the advanced analytics.
Therefore
No Big Data = Advanced Analytics