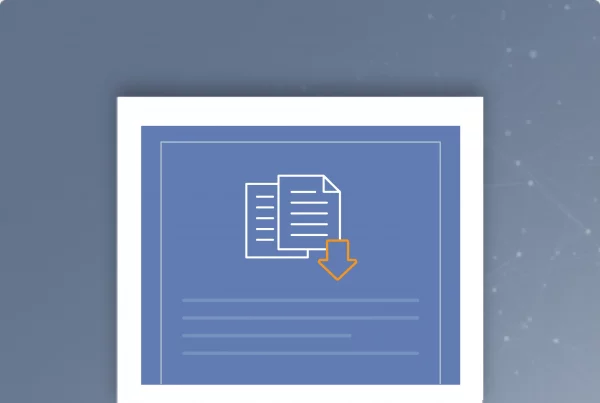
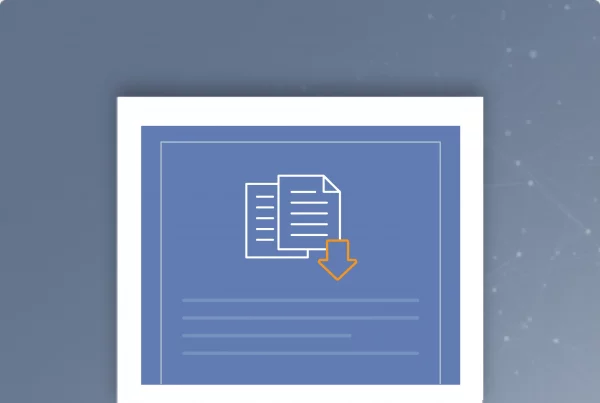
Regardless of your business needs, we will be able to provide valuable solutions that utilize unique technology combinations, methodologies, and tools that support your success.
We focus on building highly scalable and performance-centric solutions for your future needs.
With hundreds of employees spanning five countries, we have managed a multitude of data engineering and analytics projects.
Best-in-class technology partnerships with companies like Microsoft, IBM, Cloudera, MicroStrategy, Talend, Snowflake, and many more.
Sizing, Technology Selection, Design Advisory, conformed dimensions
Build Data Warehouse to get Enterprise-wide Single Version of Truth
Ongoing Maintenance of Data Warehouse
Incremental changes in DW design, index optimization
Transition to platform of your choice
Storage of any type and structure of data
Readiness Assessment, Conceptualization and Roadmap:
We help you identify where you will benefit, as well as the value-gaining steps to take.
Proof of Concept:
Our PoC Pilot programs allow you to see big data in action, helping you make crucial decisions on rollouts or expansions. We can help you plan the entire implementation, build infrastructure plans, improve design clusters, and provide team training.
Tool Evaluation:
Our extensive experience working with big data technologies has resulted in successful implementations across multiple industries and many Hadoop distributions, such as Apache, Cloudera, Hortonworks, and MapR.
Big Data Infrastructure Advisory and Planning:
We collaborate with IT and key business stakeholders to reach milestones for implementation. Our infrastructure advisory and planning services are based on the following factors: Access, Capacity, Security, Latency and Cost.
With data coming from various sources, we run multiple tests to ensure accuracy and reliability across all formats.
We ensure that all data is combined and converted to one format, compatible with that existing in data lakes and data warehouses.
We catalog the data lineage and update the data dictionary, providing better governance and access to both new and existing data.
The elastic nature of the snowflake creates scalability in the virtual warehouse, allowing you to take advantage of extra compute resources.
With traditional data warehouses, users can experience delays or failures when too many queries compete for resources. Snowflake addresses these concurrency issues with its unique multi-cluster architecture: Queries from one virtual warehouse never affect the queries from another, and each virtual warehouse can scale up or down as required. Users can efficiently obtain data without depending on other data intensive processes such as ETL/ELT loads.
Users gain the ability to combine structured and semi-structured data for analysis and load it into the cloud database, without the initial need for conversion or transformation into a fixed relational schema. Snowflake can automatically optimize how the data is stored and queried.
Snowflake’s architecture enables data sharing among Snowflake users. It also allows organizations to seamlessly share data to consumers outside of the Snowflake environment through reader accounts that can be created directly from the user interface. This functionality allows the provider to create and manage a Snowflake account for a consumer.
Snowflake is distributed across availability zones of the platform on which it runs (AWS, Azure or Google Cloud) and is designed to operate continuously, tolerating component and network failures with minimal impact to customers.
A Data Warehouse is a system for managing data that supports business insights in a meaningful way through the collection of data from varied sources.
Data warehouses have four main benefits. You can create subject oriented analysis looking at a certain area or function, there is consistency in the data accessed from different sources, the data in the warehouse is stable and won’t change, and the warehouse analyses change over time.
A cloud data warehouse is the same as a data warehouse except it uses the cloud to access and store the data. This comes with additional benefits like cost managements, ease of use, and scalability.
The main types of data warehouses are enterprise data warehouses providing support across an enterprise, operational data stores supporting reporting needs, and data marts that provide support for a specific line of business like sales.
Big data refers to large, complex growing amounts of data that cannot be handled by traditional data management solutions.
Big data is defined by data with large amounts of volume, velocity, variety, veracity, value.
Setting up big data is a complicated endeavor you have to develop a strategy to harness the data, identify important information like its sources, users, locations, and flow. You can then develop an infrastructure that will meet the analysis and computing needs of the data which is now ready to facilitate.
Big Data provides better asset management, optimizes resources, more effective strategic planning, faster turnaround time, and shorter reaction times.
Taking data from multiple sources and bringing them together to create a single source for a company to view the complete and current dataset, giving accurate access to business intelligence and data analysis.
The basis for data integration is taking data from multiple sources and making it accessible from a single location. There are multiple ways to approach this including Extract Transform Load (ETL), data virtualization, and physical data integration.
There are five main patterns of data integration:
ELT, using Extract Load Transform to move data into a repository and then convert it to be understood by the source.
Data integration is used for data governance, warehouse automation, data ingestion, data replication, marketing, internet of things, and data lake development.
Data Preparation is taking raw data cleaning and transforming it for business uses like analysis.
The benefits of data preparation are data quality, increased scalability, error detection, efficient decision making, ease in collaboration, and future proofing.
The steps are collecting data, Assessing and contextualizing data, cleaning data, formatting and enriching data, and storing data.
It is important because it allows transparency of your data for auditing, accessibility for employees of different skill sets, and repeatability so you can continually prep data with ease.